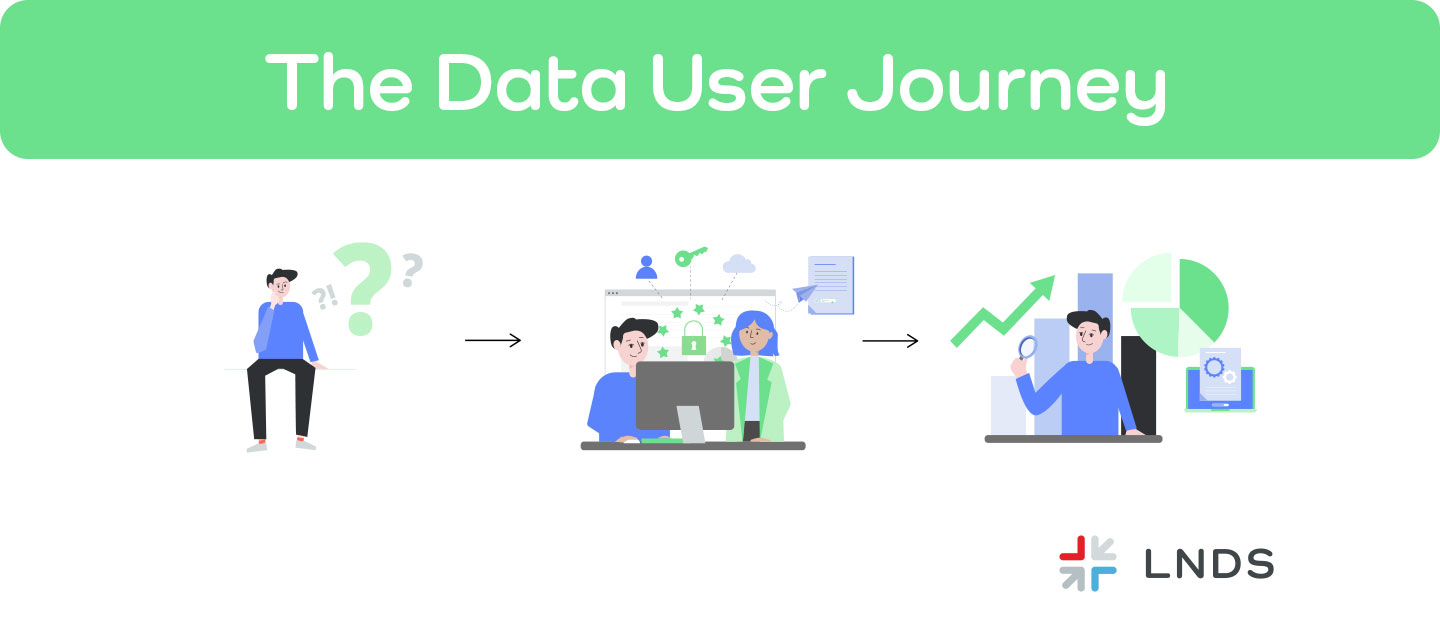
Exploring the End-To-End Data User Journey
The End-to-End Journey is a structured framework that illustrates the interactions between Data Users and Data Providers, in the context of secondary use of data. Within this framework, the Data User Journey focuses on guiding the Data Users – namely, researchers, analysts, policymakers and more – through the steps to discover, request, access, and analyse data securely and efficiently.
In our last article, we shared an introduction to the End-to-End journey, its role and impact in the data economy. In this article, we delve into the Data User Journey. We explore the seven steps of the Data User journey from ideation to resolution, illustrating how it streamlines data-driven research while ensuring compliance and security. Join us to explore this End-to-End Data User Journey, through a series of short videos, or read the full article, to see how each step of the journey brings the Data User one step closer to solving their data challenge.
To illustrate this framework, we combine the explanation of each step in the journey with an example of a Data User. We continue to follow Marc, the Data User we introduced in our last article, and whose story built the red line at the Data Summit Luxembourg 2024.
Marc has a challenge, and he needs data to get that challenge solved. Marc could be a researcher in public or private sector, or a data specialist in a company, or a policy maker in an administration. However, to keep the example simple, we call him a researcher. For Marc to obtain access to the data and making the analysis for his research, he needs to go through a process of multiple steps. The End-to-End journey guides him through this process, from defining his research challenge to securely analysing and managing the data, to finish his research.
Step 0: Ideate
Every data user’s journey begins with an idea or a new challenge. It can be a researcher who identifies a problem or hypothesis that requires data to solve, or an administration that aims to improve the services for the citizens. This initial stage involves defining the (research) question, determining objectives, and considering the types of data needed.
Example: Marc is a researcher from an energy infrastructure provider that is about to build new energy production capacity in a given area. To solve this challenge and to optimise the planning, he needs to know the correlation between the energy consumption and the geographic location, the type of households and required energy types as related factors. In this initial step, Marc refines his research question and identifies what data he will need to solve his challenge. At this step, Marc could start a Data Project with LNDS. The first step would be a meeting within the Data Project Consulting and Onboarding team, to define the project scope, goals, and anticipated impact, setting a solid foundation for a data project.
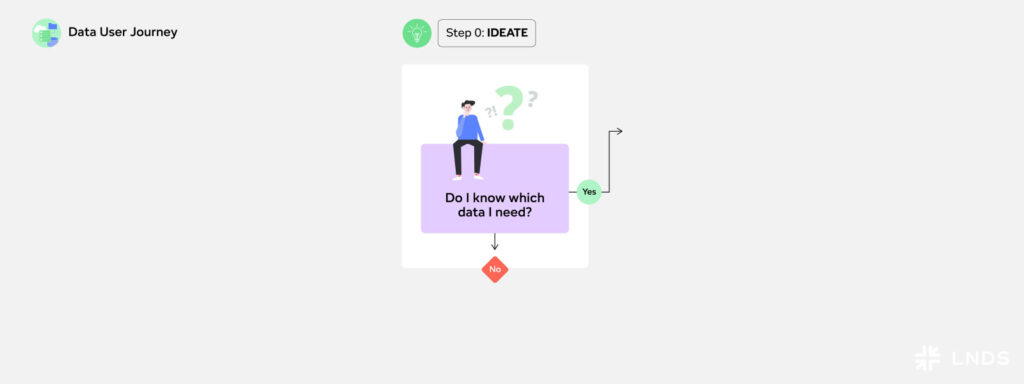
Step 1: Discover
The first step toward answering a research question or data challenge, is data discovery. In other words, how can you find the data you need? In the discovery phase, you will need to identify which datasets are available, which organisations are holding them, and what are their access conditions, data formats and standards. A data catalogue is the first place to start for discovering relevant datasets.
To find the datasets that best fit your purpose, you can further search the data catalogue by keywords, topics, file formats, descriptions, or references to prior use. In some cases, you may need to combine multiple datasets, from different sources.
Example: Marc searches data catalogues to find datasets on energy usage, household size, and geographical data, population distribution and more. To support Marc in discovering relevant data, it is important that Data Providers make their data findable through data catalogues, using standardised metadata. Standardising data and making data findable, helps researchers like Marc to move forward along his journey. One example of a data catalogue is the LNDS Data Catalogue, supporting discovery of public sector data in Luxembourg.
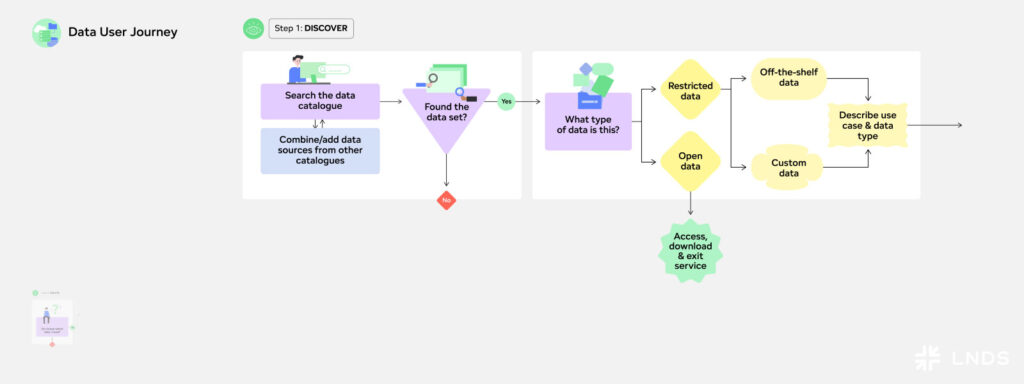
Step 2: Request Access
After identifying the necessary datasets, the next step is to formally request access from data providers. Access conditions will depend on the type of data. For open data, Data Users can simply access and download the data and continue with their research or study. However, for restricted data, the Data User needs to further describe the use case and make a formal access request.
Accessing data can become a lengthy and winded task. Different data providers, usually under different legal frameworks, often from different countries, will impose different request procedures to follow. As a Data User, you will need to understand the data use conditions and access requirements, and file your access requests via various application forms. This process may also include signing legal agreements, to prove compliance with the access request procedures.
Example: Marc has found the data he needs. In order to access the data, he needs to submit access requests to data providers. In this process, he needs to detail his research objectives and how he plans to use the data responsibly. He can only be granted access, if he is entitled to use the data. Since some datasets contain sensitive information, he may need to respond to additional questions from the data providers and sign some legal agreements, before being granted access. This can be time-consuming and complex. To support Marc in submitting and reviewing access requests, Marc could use help of the Data Access Request and Review service. With the Data Access Request and Review service, Marc can receive support in fulfilling the access application forms and how he could improve his project proposals. When he has submitted the procedures, he will need to wait for a – hopefully – positive decision.
Alternatively, if Marc wants to test his hypothesis before accessing real-world data, he can use synthetic data to simulate his research. Synthetic datasets mimic the statistical properties of real data while ensuring privacy. As the name says, the data is synthetically generated, no real data is shared. Synthetic data allows researchers to accelerate their innovation journey without waiting for approvals of accessing real data. To generate and use synthetic data, Marc can experiment and validate models in the Synthetic Data Factory, before working with actual datasets.
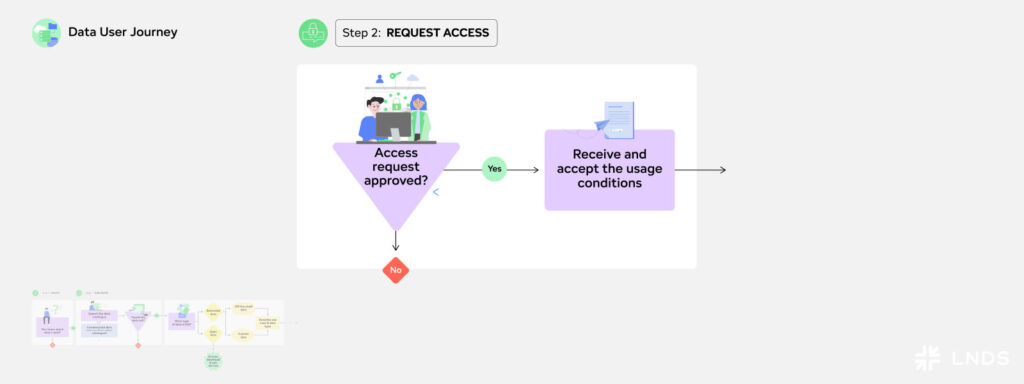
Step 3: Access
Once access is granted, the following step is to ensure a secure environment to work with the data, especially if it contains sensitive information. When a research involves sensitive data that cannot be shared, researchers will need to receive access to a Secure Processing Environment – a controlled space where data can be accessed, processed, and analysed while maintaining privacy and security.
To further protect sensitive data, pseudonymisation techniques may be applied before the data is shared in the secure processing environment. When datasets contain personally identifiable information (PII), providers must ensure that researchers do not directly handle sensitive details. Pseudonymisation is the process of replacing identifiable attributes (such as names or exact addresses) with anonymised identifiers, to reduce privacy risks while still allowing meaningful analysis. This step is to ensure that researchers work only with de-identified data while maintaining the dataset’s statistical integrity.
Example: Marc has now received all the permits to access the datasets he needs and he has signed all the necessary legal agreements. He will now gain access to a Secure Processing Environment, where he can analyse his data without compromising confidentiality. Since some datasets contain personal details, data providers first apply pseudonymisation to prevent that Marc could directly identify any individuals within his research.
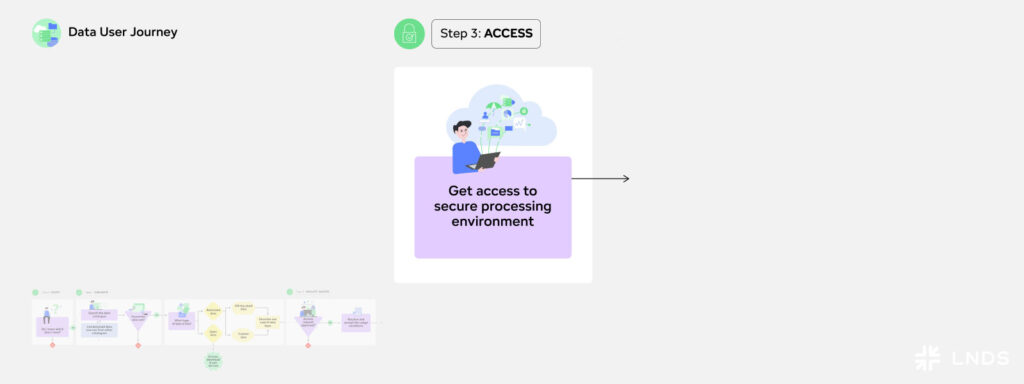
Step 4: Analyse
After having secured access to the data and the necessary tools, it is time to begin the analysis phase. In this step, the Data User can apply statistical methods, data visualisation, and advanced modelling techniques to extract meaningful insights. This step can involve merging multiple datasets, enriching data with external sources, or transforming it into a structured format suitable for analysis.
Example: Within the secure processing environment, Marc now analyses the data and works to find the answer to his research question. To support with combining datasets from different sources, clean data, or enhance its usability, he could use the Data Extraction, Enrichment, and Merging service, ensuring a high-quality foundation for analysis. Once the data is clean and clear, he will further analyse and interpret the data. With help of the Data Analysis and Visualisation Support, Marc is well equipped with tools and expertise to effectively analyse and interpret his data.
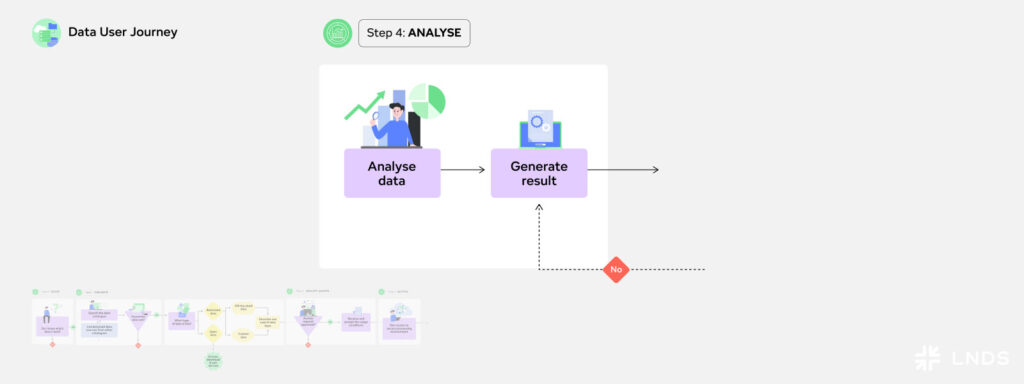
Step 5: Review Results
Before the results can be taken out of the secure processing environment, they need to be checked against data protection measures and access permits. Disclosing original data in part or in whole is usually not allowed within the access permit. The results will be reviewed by authorised reviewers, assigned by the various data providers, to ensure full compliance. The Data Providers need to be sure that data in the results are correctly anonymised, no sensitive data is shared, and all necessary approvals are ensured. This is the last step to ensure data subject privacy within the journey. After review and approval, the Data User may export the results!
Example: Before Marc can extract his results from the secure processing environment, his results need to be checked against data protection measures and access permits. Marc’s results will be reviewed by authorised reviewers, assigned by the various data providers. It is important that none of the original data is shared, neither in part nor in whole. Once Marc has the green light from the reviewers that his results contain no sensitive data, he can download his results to finish his research.
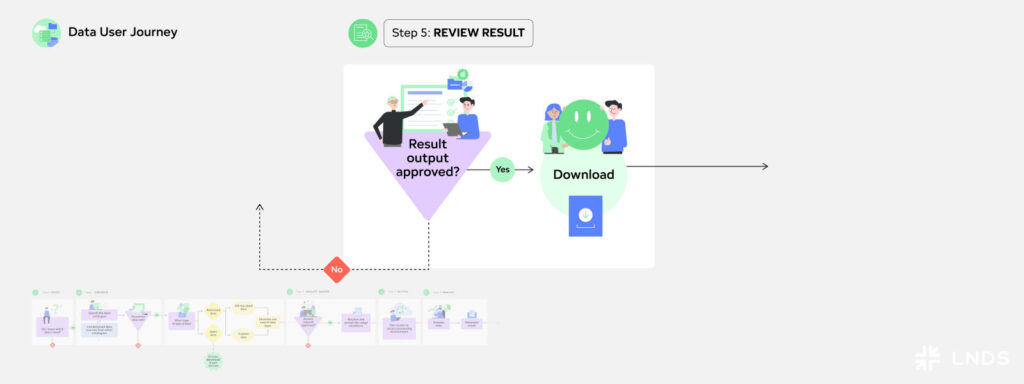
Step 6: Finish
After completing the analysis and obtaining the results and the project is finished, you need to ensure data retention policies are correctly applied. In this step the Data User needs to identify the retention obligations which could originate from legislation, institutional- or data provider policies. Depending on the policies applied, this may involve securely deleting the data, or archiving valuable results for future use. An important part of the finishing stage of the End-To-End journey, is also publishing and presenting the results.
Example: In this last step of the journey, Marc presents his research findings. He is proud to have found an optimal location for the new energy production facility present his findings to his manager. He is eager to start implementing his findings in practice, to start building the new energy production facility.
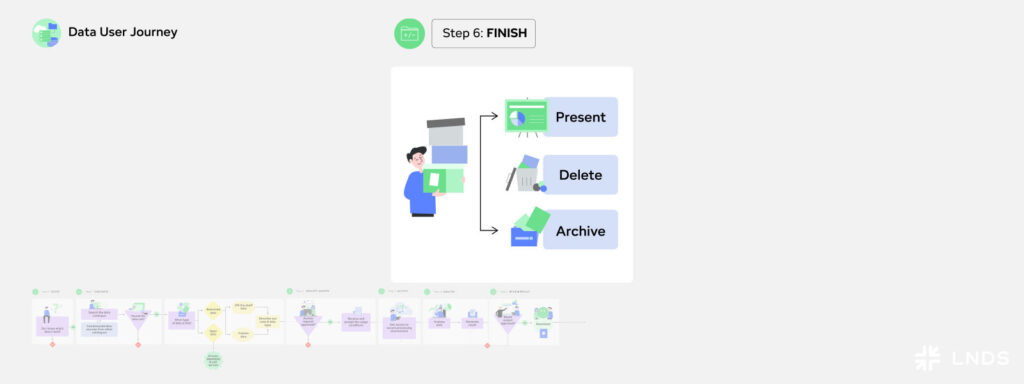
Summarising the End-to-End Data User Journey
The End-to-End Data User Journey provides a structured approach that helps researchers like Marc navigate the complexities of secondary data use. From ideation to data discovery, access, analysis, and publication, each step ensures that data is used securely, responsibly, and efficiently. By following this framework, researchers can streamline administrative processes, reduce delays, and focus on generating impactful insights. The journey also plays a critical role in ensuring compliance with ethical and legal standards, reinforcing trust between data users and providers. Throughout this journey, different data services can help researchers overcome challenges such as data access, privacy protection, and managing the data.
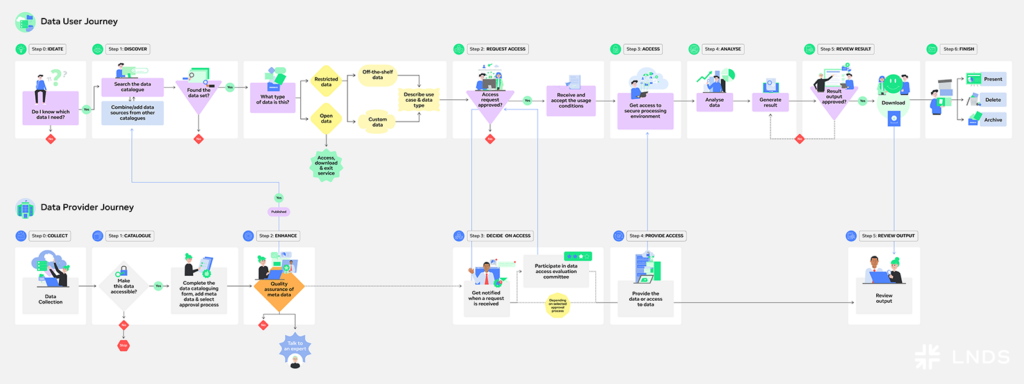
Now that we have explored the Data User Journey, we can see how researchers like Marc navigate the steps required to identify, access, and analyse data securely and efficiently. However, this process would not be possible without the crucial work done on the other side of the journey by the Data Provider.
In our next article, we will shift focus to the Data Provider Journey, exploring the key role that data providers play in preparing, structuring, and granting access to datasets. Just as researchers follow a structured workflow, data providers must also ensure that data is collected, processed, and shared in a way that meets legal, ethical, and quality standards. Together, these two journeys form the foundation of a collaborative and efficient data ecosystem. This framework helps researchers and organisations reuse valuable data responsibly to drive research and innovation.